The Power of Bayesian Network Analysis in Business
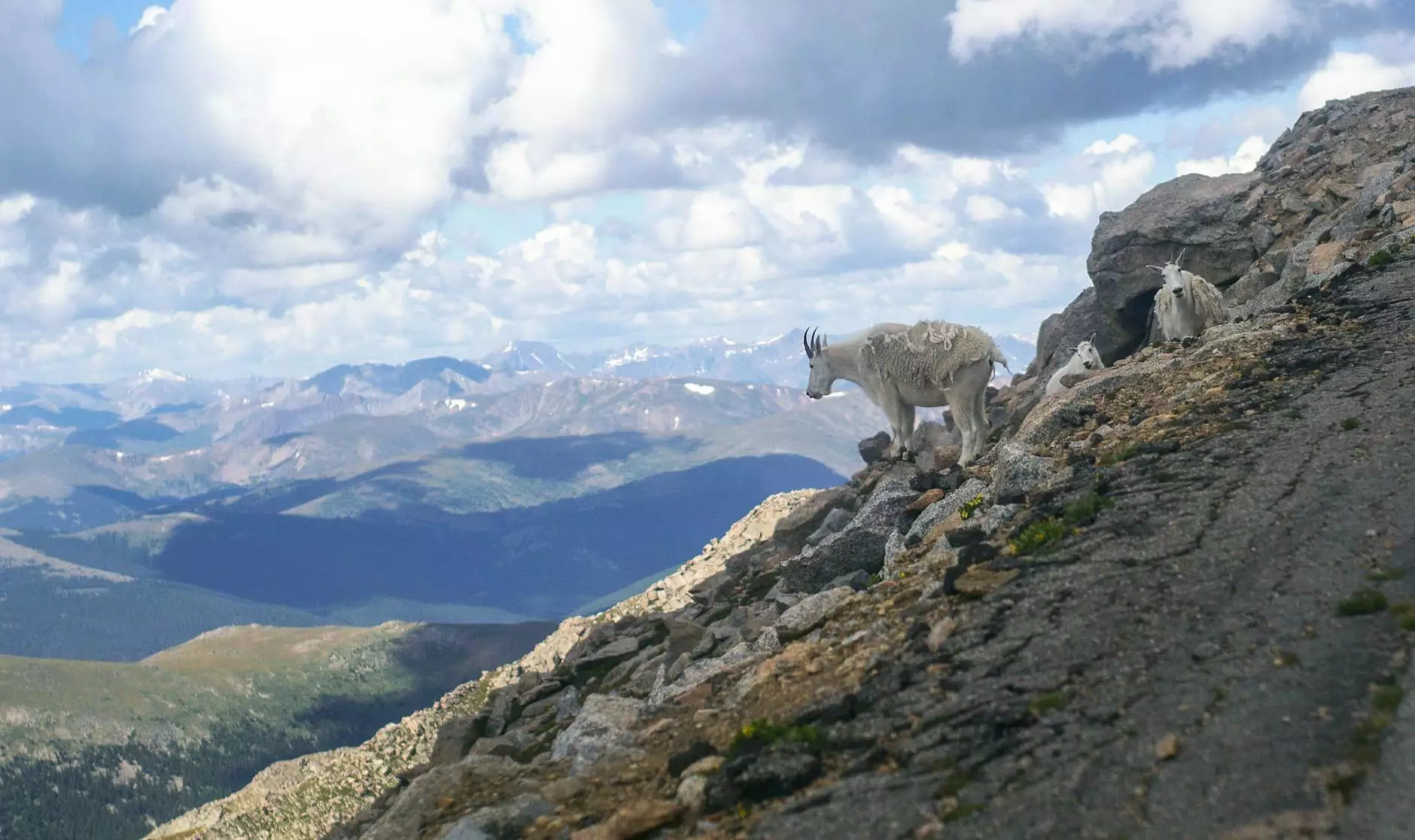
In the ever-evolving landscape of business, companies are continually seeking innovative solutions to enhance their decision-making processes and operational efficiencies. One such solution gaining traction in various industries is Bayesian Network Analysis. This sophisticated statistical method is transforming how businesses, particularly within the realms of IT Services & Computer Repair and Security Systems, analyze data and make informed decisions.
Understanding Bayesian Network Analysis
Bayesian Network Analysis is a powerful statistical tool that uses probability theory to model the relationships among a set of variables. Named after the Reverend Thomas Bayes, this method allows for the representation of probabilistic relationships among variables, capturing the influence of one variable on another effectively.
Core Concepts of Bayesian Networks
At the heart of Bayesian network analysis are directed acyclic graphs (DAGs), where:
- Nodes represent random variables.
- Edges signify the dependencies between them, indicating a direct influence.
These structures enable businesses to visualize and quantify uncertainty, leading to more informed choices based on empirical data.
Why Bayesian Network Analysis is Essential for Businesses
For businesses focusing on IT Services & Computer Repair and Security Systems, Bayesian Network Analysis provides several advantages:
Enhanced Decision Making
By utilizing Bayesian networks, companies can make smarter decisions based on predictive analytics. The models allow businesses to incorporate prior knowledge—be it historical data or expert opinion—into their analysis, which can significantly enhance the decision-making process. For instance:
- IT Services: Companies can predict system failures by analyzing the relationships between various components and their performance metrics.
- Security Systems: Organizations can assess risks by examining the likelihood of potential threats and vulnerabilities in their systems.
Improved Risk Assessment
In the security domain, risk assessment is critical. Bayesian networks can provide detailed insights into potential threats by evaluating a multitude of variables, such as:
- The probability of different types of attacks.
- The potential impact of these attacks on the organization's operations.
This approach not only helps in preparing for potential risks but also in implementing effective mitigation strategies tailored to specific vulnerabilities.
Real-World Applications of Bayesian Network Analysis
To truly appreciate the strength of Bayesian Network Analysis, it is beneficial to look at real-world applications across the two categories of interest—IT Services & Computer Repair and Security Systems.
IT Services & Computer Repair
In the field of IT services, Bayesian network analysis can assist in predictive maintenance, which is paramount for reducing downtime. Consider the following applications:
1. Predictive Maintenance
By analyzing the historical performance data of hardware components, businesses can predict failures before they occur. For example:
- Analyzing disk failure data can inform IT departments about which servers might need replacement or repair soon.
- Understanding environmental conditions (like temperature and humidity) and their effects on hardware performance can guide preventive actions.
2. Customer Support Optimization
Using Bayesian methods, businesses can enhance their customer support systems by predicting customer needs based on past interactions. This leads to:
- More personalized service.
- Efficient resource allocation, ensuring skilled technicians are available for high-probability issues.
Security Systems
In the realm of security systems, applying Bayesian network analysis can significantly bolster threat detection and risk management practices:
1. Intrusion Detection Systems
Bayesian networks can improve the effectiveness of intrusion detection systems by correlating different types of network activities. For example:
- Identifying abnormal behaviors by analyzing user access patterns and flagging anomalies.
- Integrating threat intelligence data to assess the likelihood of an attack in real-time.
2. Vulnerability Management
Organizations can use Bayesian analysis to prioritize vulnerabilities based on the likelihood of exploitation. This means:
- Resources can be focused on remediating the most dangerous vulnerabilities first.
- Limited resources are effectively allocated towards the highest risks.
Implementing Bayesian Network Analysis
Implementing Bayesian Network Analysis within a business requires a strategic approach. Below are the essential steps that organizations should consider:
1. Defining the Problem
Clearly articulate the business problem at hand. What decision needs to be made? What uncertainties are involved?
2. Data Collection
Gather relevant data that will inform the model. This could include:
- Historical operational data.
- Expert insights.
- Market analysis reports.
3. Model Construction
Create a Bayesian network model. This involves defining nodes and edges based on the collected data and insights. Tools such as Netica, GeNIe, or even programming languages like Python (using libraries such as pgmpy) can be employed to create these models.
4. Analysis & Interpretation
Once the model is created, perform sensitivity analysis to understand how changes in one variable may affect others and interpret the results to draw actionable insights.
5. Decision Making
Utilize the insights gleaned from the analysis to make informed decisions. Implement changes based on model predictions and continuously monitor outcomes.
Challenges in Bayesian Network Analysis
Despite its numerous benefits, Bayesian Network Analysis is not without its challenges:
1. Complexity in Model Construction
The construction of a Bayesian network can be complex, especially for larger systems with numerous interdependencies.
2. Data Requirements
High-quality, relevant data is essential for accurate modeling. Poor quality data can lead to misleading results.
3. Interpretability
Understanding and interpreting the outcomes of Bayesian models requires a certain level of expertise, which may not be readily available within all organizations.
Conclusion
In conclusion, the application of Bayesian Network Analysis in businesses, especially within IT Services & Computer Repair and Security Systems, presents an invaluable opportunity for enhancing decision-making processes and operational efficiency. As businesses continuously strive for a competitive edge in today's data-driven world, integrating Bayesian network models can prove to be a significant leap forward in harnessing the power of data. By investing in training and resources to effectively implement these models, companies can unlock new levels of productivity and security while expertly navigating the complexities of their operating environments.
As we move further into the age of information, those who embrace tools like Bayesian Network Analysis will undoubtedly lead the charge towards smarter and more resilient business practices.