Unlocking the Potential of Medical Datasets for Machine Learning
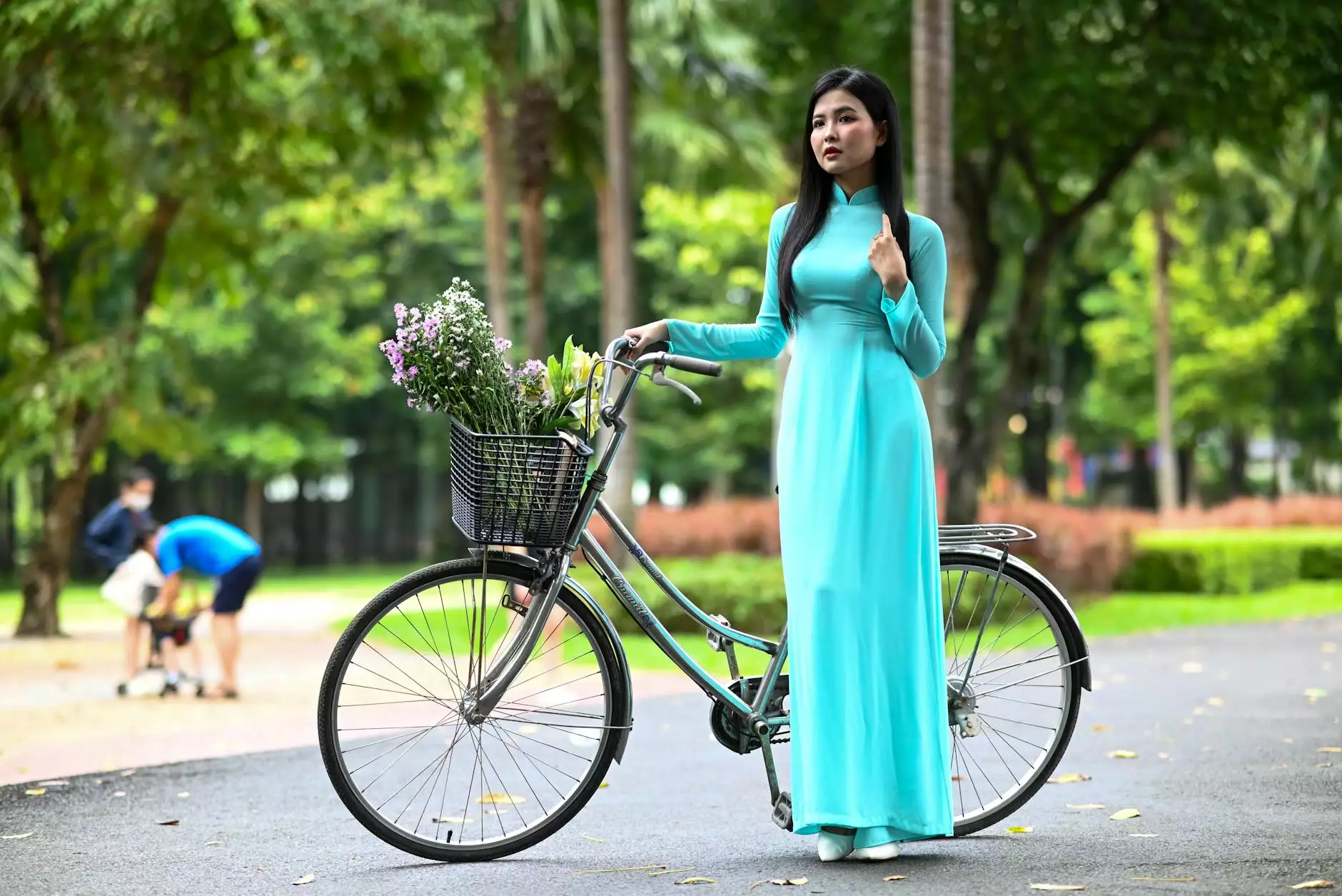
In the age of digital transformation, machine learning (ML) has emerged as a cornerstone in various industries, particularly in healthcare. The intersection of medical datasets and machine learning is creating unprecedented opportunities for innovation. This article delves into the multitude of ways that medical datasets empower machine learning applications, enhancing patient care and advancing medical research.
What is a Medical Dataset?
A medical dataset comprises a collection of medical-related data. This data can include patient demographics, clinical evaluations, diagnostic reports, medical imaging, genomic data, and more. The goal is to gather structured and unstructured data that can be utilized for analysis.
- Clinical Data: Information derived from patient visits, treatments, and outcomes.
- Genomic Data: Genetic and molecular data that provide insights into hereditary conditions.
- Imaging Data: Images produced by various imaging techniques such as MRI, CT scans, etc.
- Electronic Health Records (EHR): Comprehensive records of patients' medical histories.
The Role of Medical Datasets in Machine Learning
Machine learning algorithms thrive on data. The richer and more diverse the dataset, the better the algorithm's performance. Medical datasets present unique challenges and opportunities:
1. Enhancing Diagnostic Accuracy
Utilizing a medical dataset for machine learning enables healthcare providers to enhance diagnostic accuracy. Machine learning models can detect patterns in data that may not be immediately apparent to clinicians. For example:
- Predictive Analytics: ML algorithms can predict disease occurrence based on historical data.
- Image Recognition: Advanced image recognition techniques can identify anomalies in medical imaging.
By training models on large datasets, healthcare systems can implement better diagnostic tools that assist physicians in making informed decisions.
2. Personalizing Patient Treatment
Every patient is unique, and so is their response to treatment. Machine learning, powered by medical datasets, facilitates personalized medicine, tailoring treatment plans that best fit individual patients. This leads to improved outcomes and patient satisfaction.
3. Streamlining Healthcare Operations
Data-driven insights allow healthcare organizations to optimize operations. Machine learning applications can predict patient inflow, manage staffing levels, and enhance resource allocation, thus reducing operational costs.
4. Drug Discovery and Development
The pharmaceutical industry heavily relies on machine learning for drug discovery. By leveraging extensive medical datasets, researchers can identify potential drug candidates faster than traditional methods. The phases of drug development can be significantly accelerated through:
- Data Mining: Extracting valuable information from large volumes of health data.
- Modeling Biological Processes: Using machine learning to simulate how drugs interact with biological systems.
Machine Learning Techniques in Analyzing Medical Datasets
Various machine learning techniques are employed to analyze medical datasets, each with distinct advantages. Below are some common techniques:
1. Supervised Learning
In supervised learning, algorithms are trained on a labeled dataset, where the outcome is known. This method is extensively used in predictive analytics, where the goal is to forecast future medical events, such as:
- Diagnosing diseases: Training on past patient data to predict future diagnoses.
- Treatment Predictions: Suggesting treatment plans based on historical patient data.
2. Unsupervised Learning
Unsupervised learning deals with data that is not labeled. It's primarily used for clustering and association tasks. For example:
- Identifying Patient Groups: Classifying patients based on similarities in health metrics.
- Detecting Anomalies: Recognizing unusual patterns in data, which may indicate potential health issues.
3. Deep Learning
Deep learning, a subset of machine learning, utilizes neural networks with many layers. It's particularly effective for processing complex datasets, like images. Applications in healthcare include:
- Medical Image Analysis: Automating the detection of tumors or fractures in imaging tests.
- Natural Language Processing: Analyzing clinical notes and other unstructured data for valuable insights.
Challenges of Using Medical Datasets in Machine Learning
Despite the potential of medical datasets for machine learning, challenges do exist:
1. Data Privacy Concerns
Healthcare data is sensitive, and protecting patient privacy is paramount. Compliance with regulations like HIPAA (Health Insurance Portability and Accountability Act) is essential to ensure confidentiality when utilizing medical datasets.
2. Data Quality and Standardization
Medical data can be inconsistent and fragmented. Ensuring data quality through proper collection and standardization processes is crucial for achieving reliable outcomes in machine learning applications.
3. The Need for Interdisciplinary Collaboration
Effective machine learning applications involve collaboration between data scientists, healthcare professionals, and domain experts to translate data insights into practical solutions.
The Future of Medical Datasets and Machine Learning
The future of medical datasets for machine learning is promising, characterized by continuous advancements in technology. Some trends to watch include:
- Integrating Wearable Technology: Collecting real-time health data from devices to enhance medical research and patient monitoring.
- Advancements in AI: Developing more sophisticated algorithms that can analyze data efficiently and accurately.
- Collaboration Across Institutions: Increasing partnerships between healthcare providers, tech companies, and research institutions.
Conclusion
In conclusion, the role of medical datasets in machine learning is vital to transforming healthcare. By harnessing the power of data, we can enhance diagnostic accuracy, personalize treatments, streamline operations, and innovate drug discovery. As we continue to develop robust algorithms and embrace technological advancements, the future of healthcare looks bright.
For businesses in the Home Services and Keys & Locksmiths industries, leveraging the power of medical datasets can open avenues for improvement in customer services and operational efficiencies. Remaining current with technology trends can lead to significant advancements, allowing businesses to stay competitive in a rapidly evolving marketplace.
Explore the possibilities and embrace the shift towards data-driven strategies today!